Unlocking the Power of Vector Databases: A Beginner’s Guide
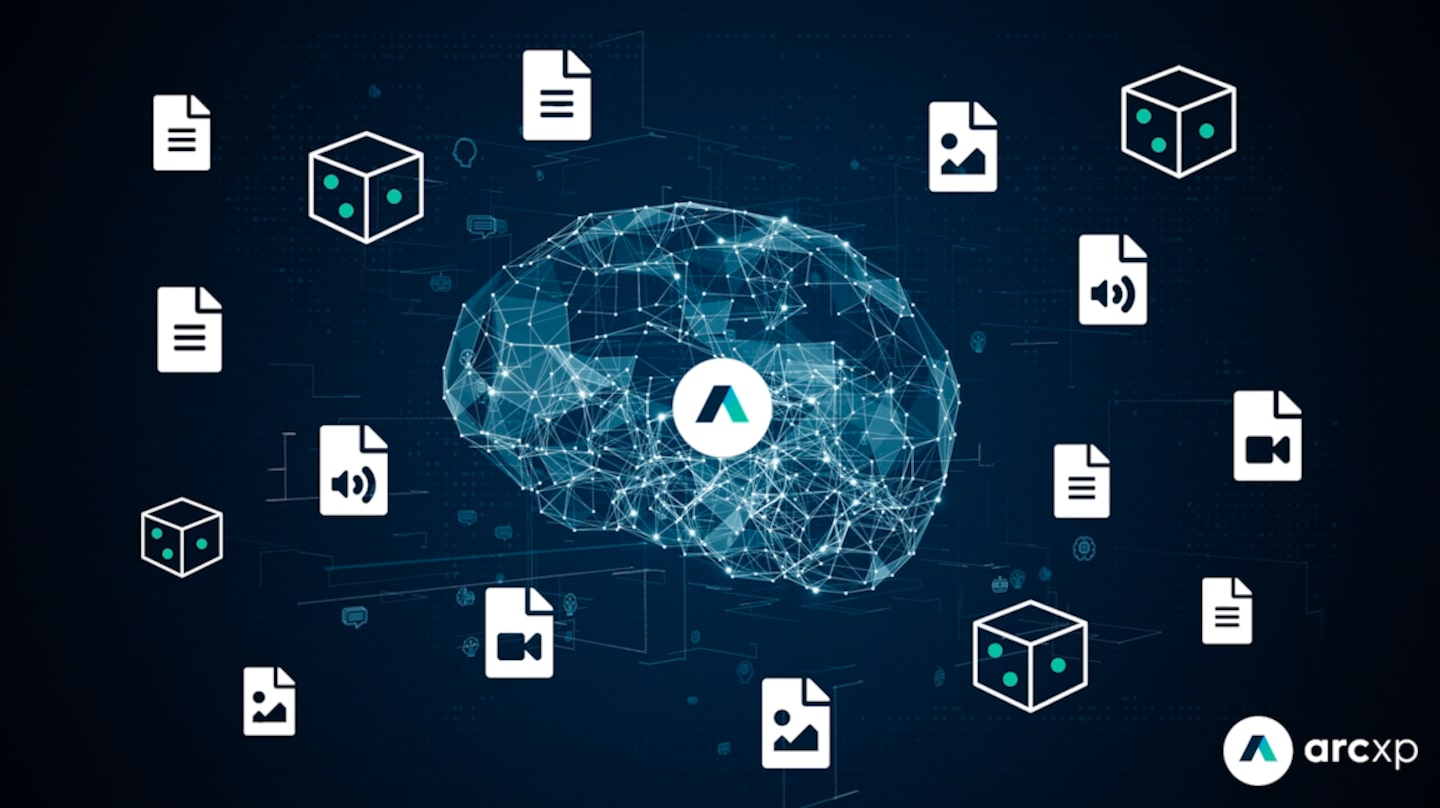
Imagine searching for something online and wishing the search engine could understand your intent, even if you don’t have all the information you need. Perhaps you’re looking for a colleague’s article written months ago, but you can’t remember the exact details. Traditional search engines might fall short in these scenarios, but this is where vector databases come into play. As an essential component of AI innovation in the media industry, vector databases are transforming how content is searched, created, and personalized.
What is Vector Search?
Vector search enables you to search by meaning rather than just keywords. Unlike traditional search engines that rely on exact matches, vector search understands the context of your queries. This is particularly useful when you’re unsure of the specific terms to use or when you’re searching for something abstract or complex.
How Vector Databases Work
Vector databases add a layer of semantic understanding to your data, documents, or text. This means they can power recommendation engines or search functions in a way that’s more aligned with human thinking.
To put it simply: Traditional databases, like relational databases, use rows and columns to organize data, and document databases use collections and documents. Vector databases, on the other hand, use arrays of numerical values—called vector embeddings. These embeddings capture the essence of the information in a form that AI can understand, like a digital summary of the content.
The Advantage of Vector Search
When you perform a vector search, you’re not just searching for exact keyword matches. Instead, the search engine looks for contextual similarities between the data points, using a process called “nearest neighbor search” across multiple dimensions. This allows AI to make connections between seemingly unrelated pieces of information, almost like drawing a map that shows how different pieces of content are related.
For example, if you have stories about Mexico and dogs, a traditional database might not see any connection between them. But a vector database, with its ability to understand context, might recognize that both stories could be about travel or lifestyle, depending on the content.
Why This Matters for Media Organizations
As media organizations increasingly integrate generative AI into their processes, vector databases become a powerful tool for enhancing content creation, curation, and personalization.
- Content Creation: With vector databases, generative AI can better understand the relationships and context within your content library. For example, if you’re producing an article on climate change, the AI application could automatically suggest relevant data, quotes, or past articles that align with the topic, even if they don’t share the exact keywords. This results in more comprehensive and contextually rich content.
- Content Curation: Vector databases enable more sophisticated content curation, helping editors and AI tools to recommend articles, videos, or multimedia that are thematically related. This is particularly useful for creating personalized user experiences, where the content is tailored to an individual’s interests, even if those interests aren’t explicitly stated.
- Content Personalization: By understanding user behavior and preferences through the context of their interactions, media organizations can use vector databases to offer more relevant and engaging content. Whether it’s suggesting related stories or tailoring newsletters, the ability to understand and predict what a user might find interesting goes beyond simple keyword matching.
- Efficient Search for Archives: Media organizations often have vast archives of content. With vector search, journalists and editors can quickly find relevant information, even if they don’t remember the exact details. This speeds up the research process and ensures that valuable content is easily accessible, enhancing the overall quality of new productions.
Real-World Applications
Several solutions today incorporate vector search capabilities, such as MongoDB with Vector Atlas Search, PGVector, Pinecone, and Weaviate. These tools allow businesses to harness vector databases for faster, more accurate, and context-aware searches.
This technology is more than just a search upgrade—it’s a fundamental shift in how we interact with data. By enabling machines to understand and recommend based on context and meaning, businesses can dramatically improve the efficiency and relevance of their search functions and recommendation engines.
At Arc XP, vector databases are set to enhance our AI capabilities, particularly with our Arc Intelligence suite. By using vector databases, we’re pushing the boundaries of what AI can do, helping businesses not only store and manage data but also unlock its full potential.
Vector databases act like an AI-powered brain for your content, making connections that would otherwise be impossible. As this technology continues to evolve, it will become an essential tool for any business looking to stay ahead in the digital landscape.
Visit our AI with Arc XP page to stay updated on cutting-edge AI innovations in the media industry and see how leading organizations are integrating these advancements into their strategies.
Recent resources
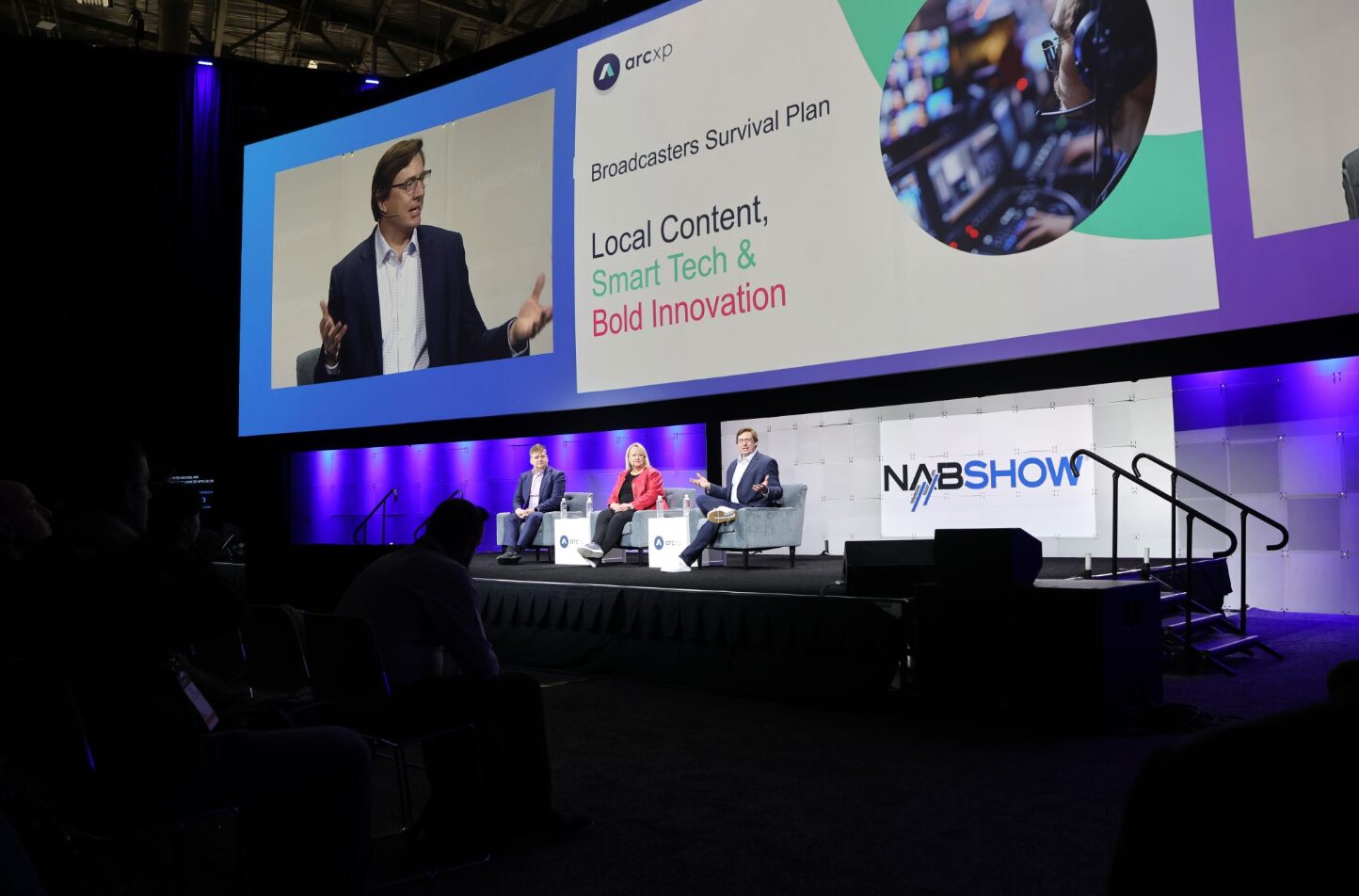
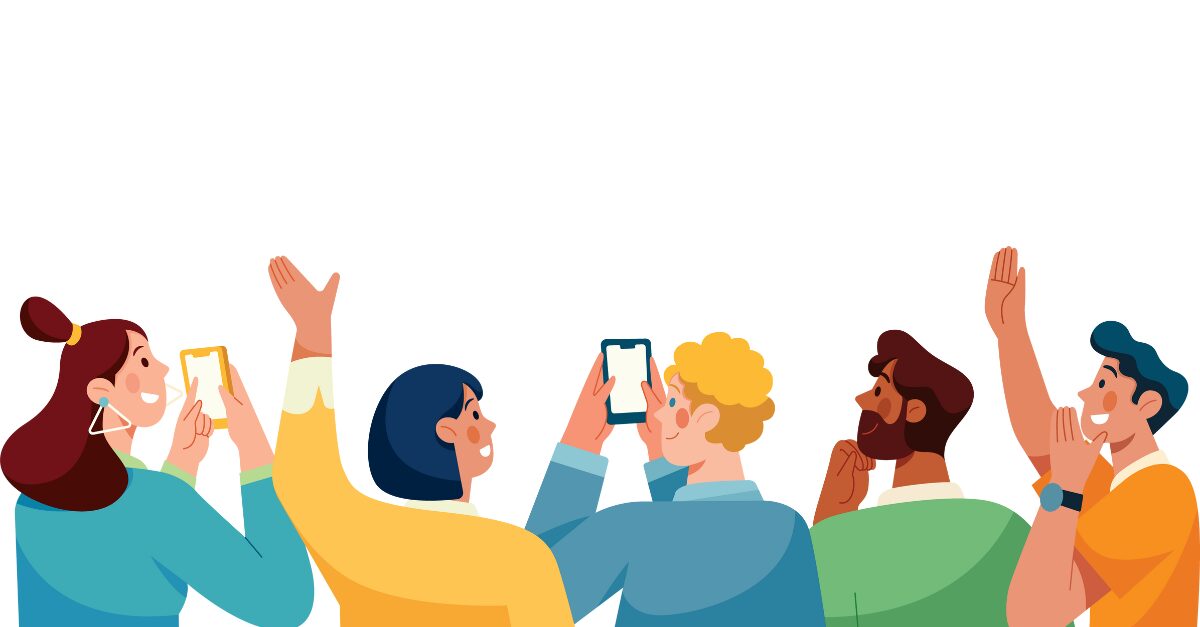
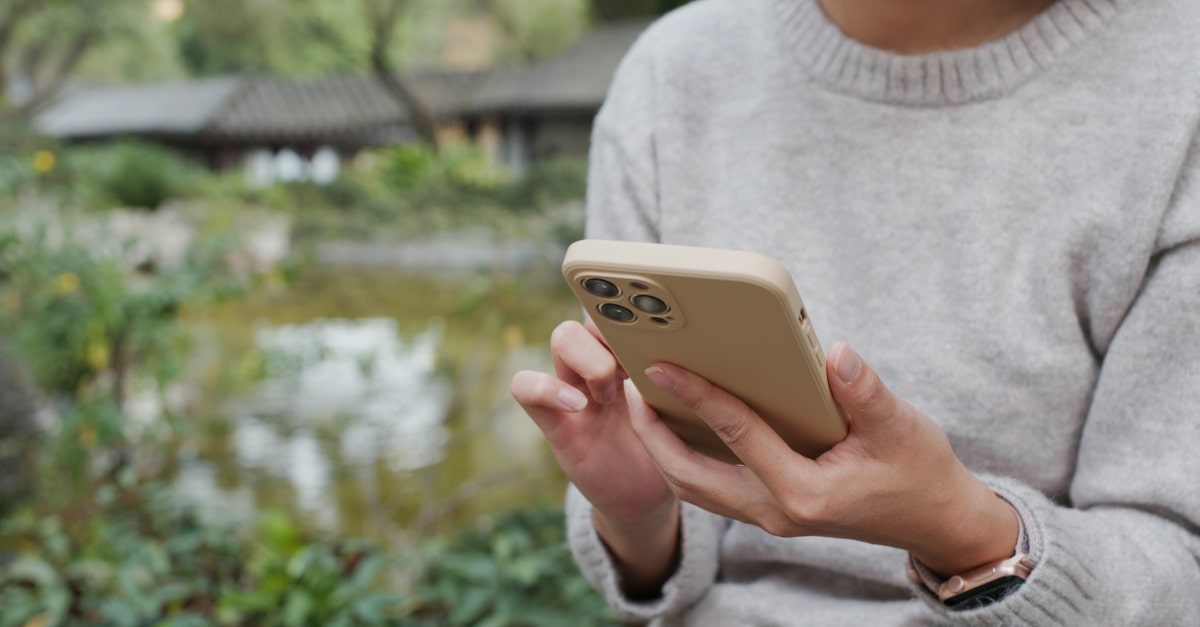
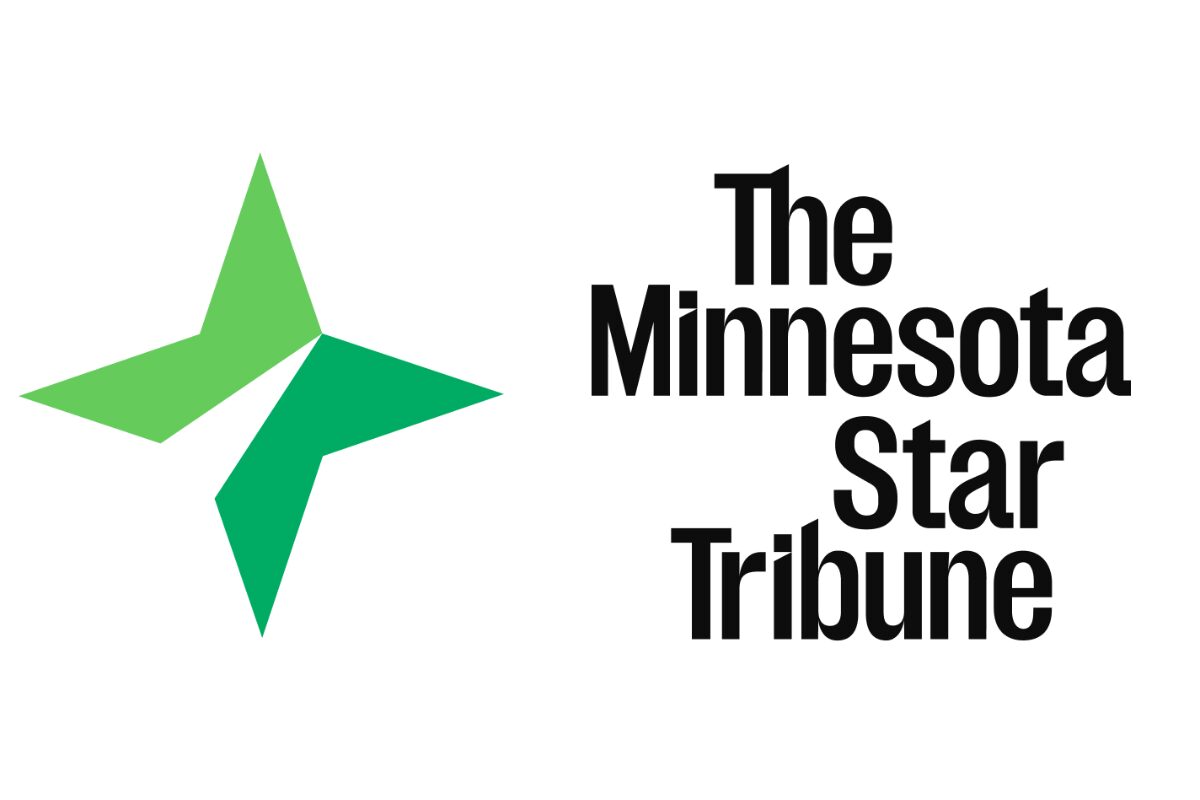